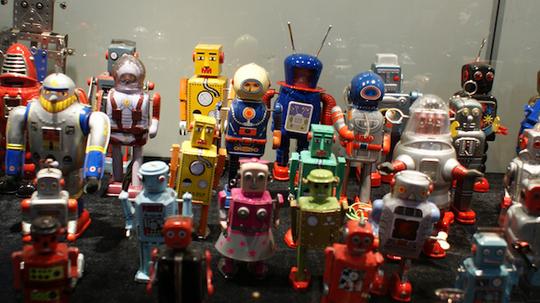
As devices become limb extensions, and our interactions with technology get more complex, we are faced with a pertinent question: Are devices good at understanding humans?
Today, humans are building mechanical substitutes for everything from driving cars to making dinner. And yes, machines are getting more intelligent, but how do we get them to be more intuitive? How do we teach devices to understand implicit emotions instead of explicit intentions? And can we trust devices to make the optimal decision for us when we cannot?
All these questions are at the forefront of emotional AI research. BostInno spoke to Taniya Mishra, Director of AI Research and speech scientist at Affectiva, an MIT Media Lab company that develops software to recognize human emotions. Below is an edited excerpt of the interview:
Can you explain Affectiva’s technology and founding principle?
In a nutshell, Affectiva's mission is to humanize technology by bringing emotion AI solution that can read people's emotions and cognitive states like another human being would by observing their face and listening to their voice and to do it in real time and do it with context with mind.
Why do you think that’s a crucial area of study right now?
Human-computer interaction right now is very transactional because the voice command applications today don’t understand the implicit emotional states and humans are not great at talking about how we feel, we are better at expressing intentions. And this is key when we think about what we want our devices to do. For example, if we are angry or frustrated or nervous while driving, how do we want our cars to respond to our changing emotional state.
How is Affectiva working on this problem?
We take a data-driven deep learning approach to detect emotions from face or voice and then we do an end-to-end learning model which means we feed the audio and video clips to the software to make it perceive emotions through behavioral cues. Before this model, the prior approach was to take audio and extract the features out of it like stress pattern, silent pattern, tone, pitch etc. and then feed these features to the model. We are building a platform that would teach the system to perceive and analyze this.
The cost of error or reading implicit emotions incorrectly can be high. How do you correct for this?
We think about the idea of cost-based optimization carefully. We are measuring things like false positives and false negatives constantly and teaching the model the difference between the two. We are always analyzing the kind of errors that could take place and the solution is to be conservative in predicting that and then fine-tuning our model to minimize errors. For example, if your car looks at facial cues and interprets your state of mind as being frustrated or nervous, it might ask you to breathe deeply or if you are drowsy, it might ask you to pull over at a rest-stop. Of course, sometimes the system can be inaccurate but it’s crucial to account for worst-case scenarios and build in responses accordingly.
I suspect privacy being a concern for some of this?
Absolutely, all data we collect is opt-in, as emotions are private and should not be recorded volcally if we don’t want it. Which is why, we do not work with the surveillance industry as a principle. Additionally, we build models that run on the cloud and on-device, which has no connection back to our servers in anyway. We run thorough assessments of whether or not we believe the use case is an ethical use of our technology, as well as if the interested party would require explicit consent, or if there is implicit consent.
What verticals do you adopting this in a big way?
We see a lot of intererest from the automotive sector, robotics, market research companies (a big revenue generator) and education.
What is the skillset you would look for in prospective hires?
Affectiva is hiring and we look for people with coding, machine learning, speech processing skills.